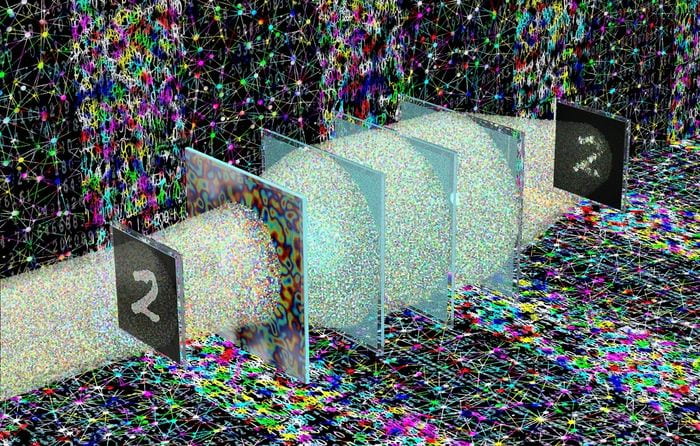
Seeing through random diffusers instantly without a computer
Imaging through scattering and diffusive media has been a challenge for many decades, with numerous solutions reported so far. In principle, images distorted by random diffusers (such as frosted glass) can be recovered using a computer. However, existing methods rely on sophisticated algorithms and codes running on computers that digitally process the distorted images to correct them.
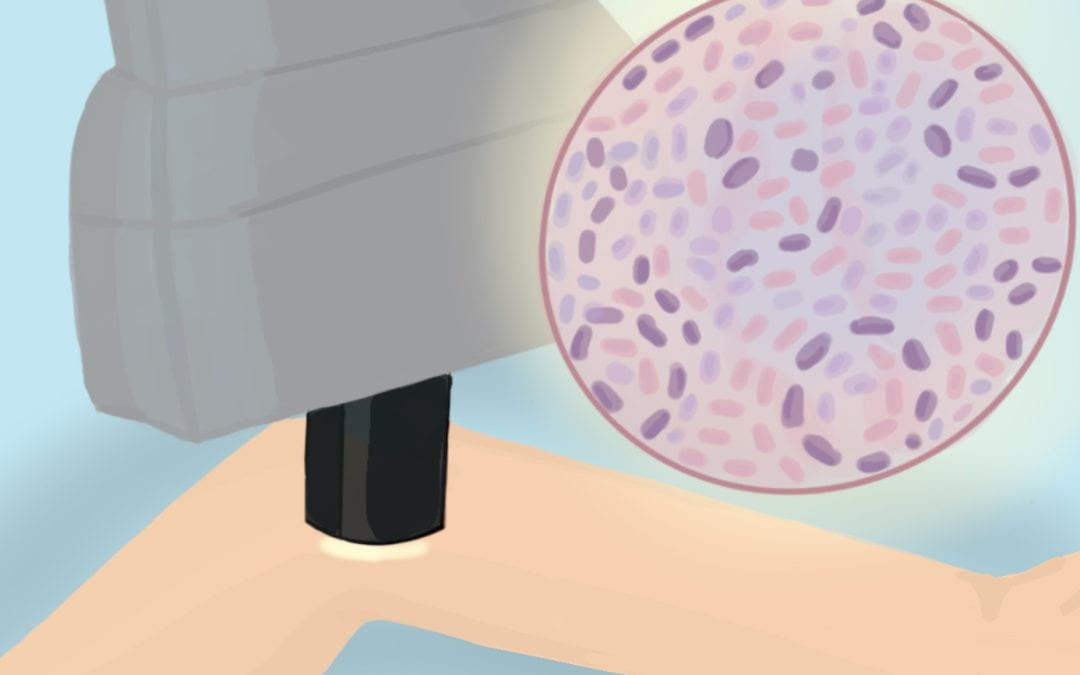
UCLA researchers streamline skin tissue analysis with virtual staining technique
UCLA researchers have developed a virtual, non-invasive method of visualizing skin tissue that could transform how skin diseases are diagnosed.
In a Nov. 18 study, UCLA researchers described how artificial intelligence could be used to transform a black-and-white image of the skin obtained by a non-invasive microscope into a colorized, highly-detailed image, similar to one obtained through a biopsy.
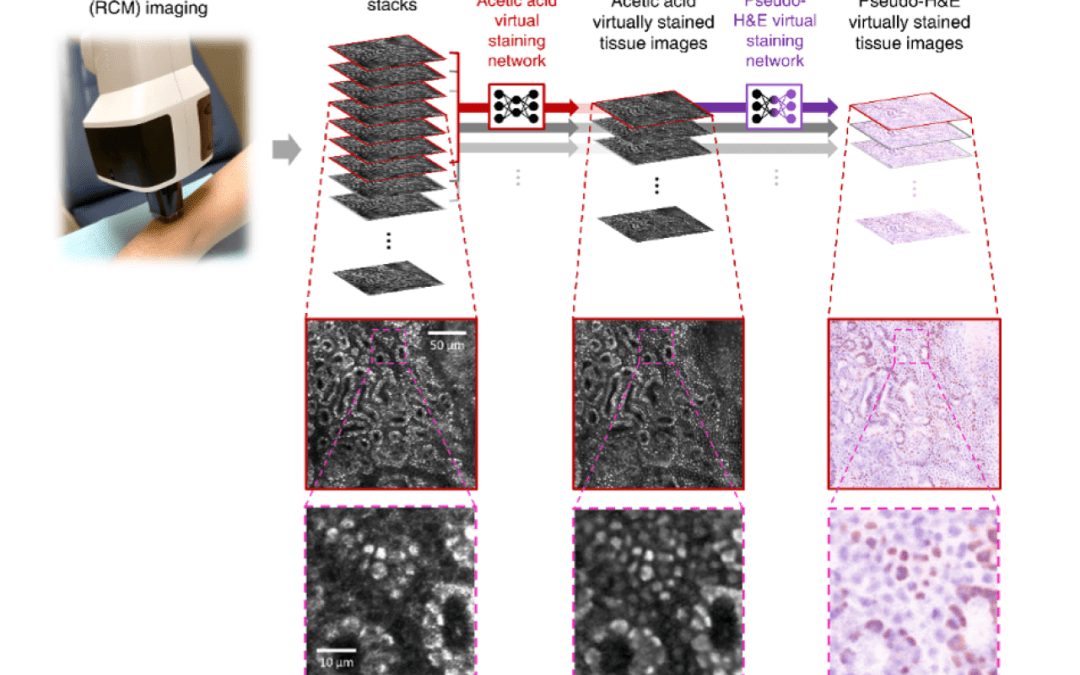
AI Imaging Method Provides Biopsy-free Skin Diagnosis
A deep learning-enabled imaging technology, developed by UCLA professor Aydogan Ozcan and colleagues, provides a noninvasive way to rapidly diagnose skin tumors, allowing earlier diagnosis of skin cancer. The technology bypasses reliance on skin biopsies, which are invasive, cumbersome, and time-consuming. It can take days to receive the results of a biopsy.
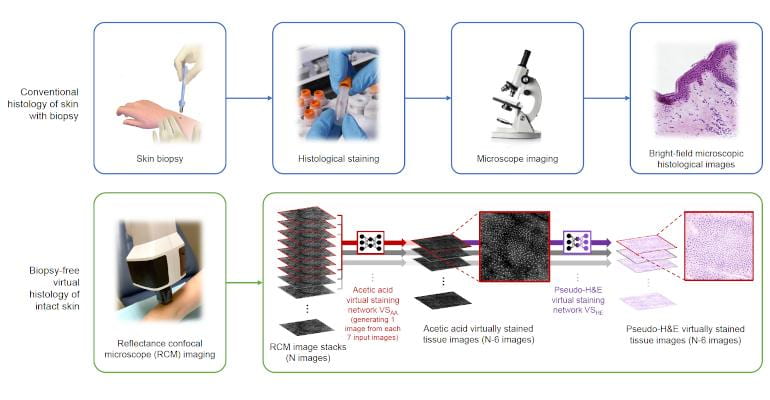
Can This New Tech Reduce the Need for Skin Biopsies?
Typically, when diagnosing a skin tumor, a biopsy is needed to extract tissue that is later sectioned into thin layers, stained, and examined under a microscope for abnormalities in a process called histology. However, biopsies are invasive, leave scars, and might end up being unnecessary.
“But what if we could entirely bypass the biopsy process and perform histology-quality staining without taking tissue and processing tissue in a noninvasive way? Can we create images that diagnosticians can benefit from?” wondered Dr. Aydogan Ozcan, Chancellor’s Professor and Volgenau Chair for Engineering Innovation, UCLA Samueli School of Engineering, in an interview with MD+DI.
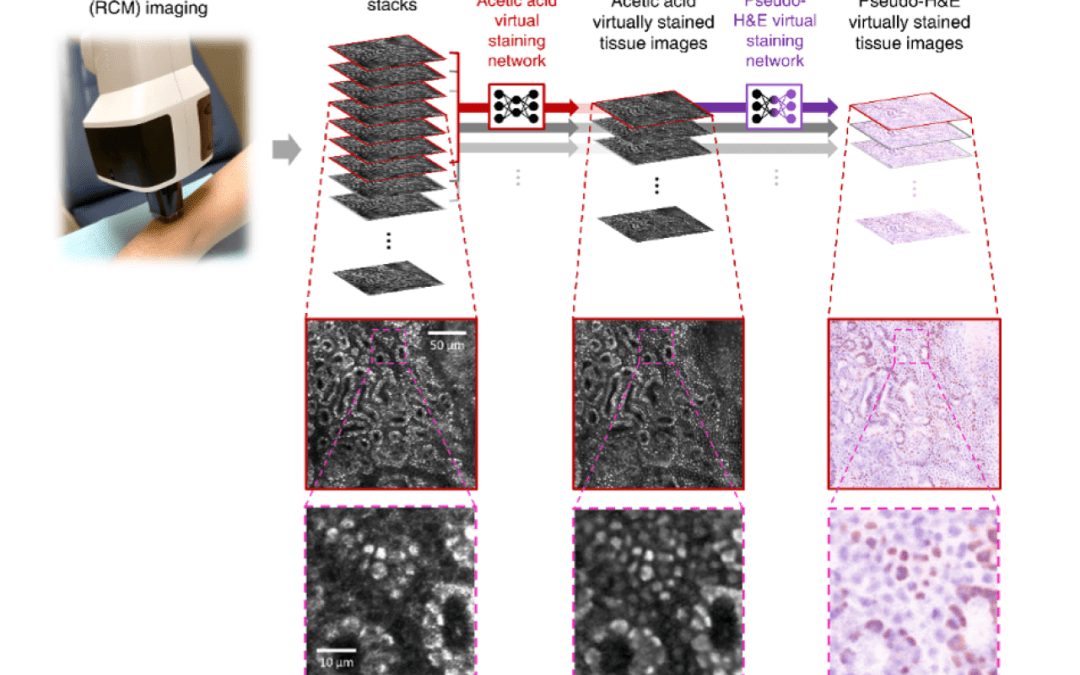
AI Imaging Method Provides Biopsy-free Skin Diagnosis
A deep learning-enabled imaging technology, developed by UCLA professor Aydogan Ozcan and colleagues, provides a noninvasive way to rapidly diagnose skin tumors, allowing earlier diagnosis of skin cancer. The technology bypasses reliance on skin biopsies, which are invasive, cumbersome, and time-consuming. It can take days to receive the results of a biopsy.
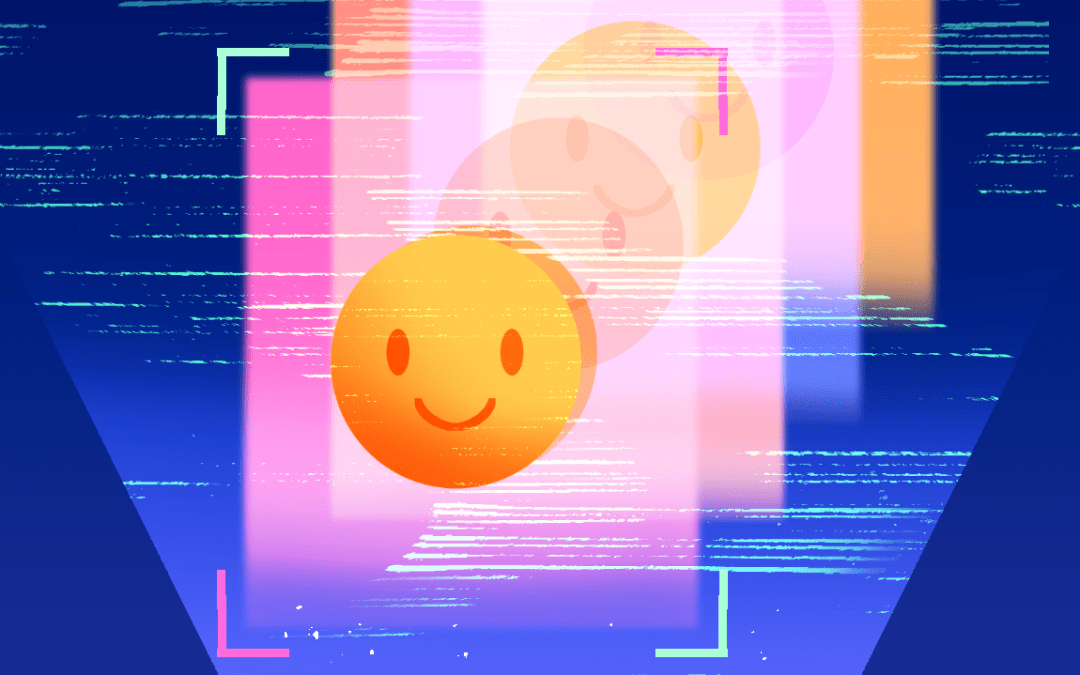
Researchers at UCLA develop computer-free technology for deciphering holograms
UCLA researchers developed a computer-free system that can instantaneously reconstruct holograms, helping further technology in the field of optical processing, according to an Oct. 27 study.
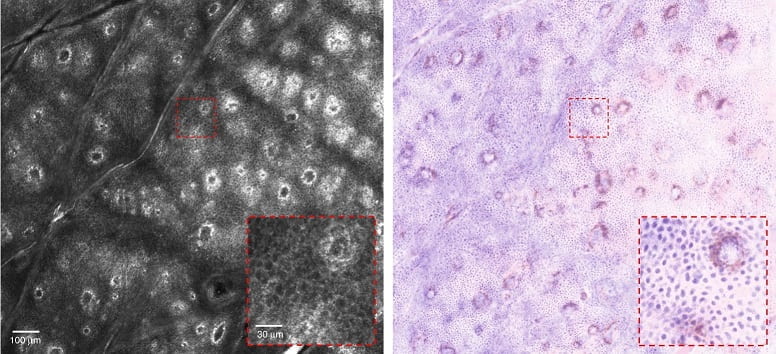
Virtual staining set to reduce need for skin biopsies
Deep learning transforms confocal microscopy images of unstained skin into stained images showing tissue structure.
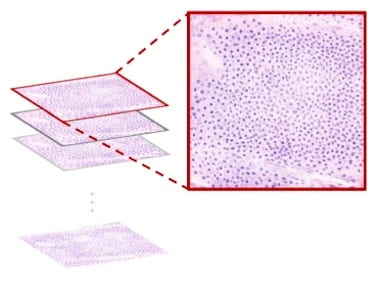
UCLA deep-learning reduces need for invasive biopsies
A project from the lab of Aydogan Ozcan at UCLA has now made a new breakthrough in the data processing dimension, developing a deep-learning framework to transform non-invasive confocal microscopy data from unstained skin into virtually-stained images.
Reported in Light Science & Applications, the new framework may permit more rapid diagnosis of malignant skin neoplasms and reduce invasive skin biopsies, in line with the current clinical drive towards diagnosing skin disease non-invasively.
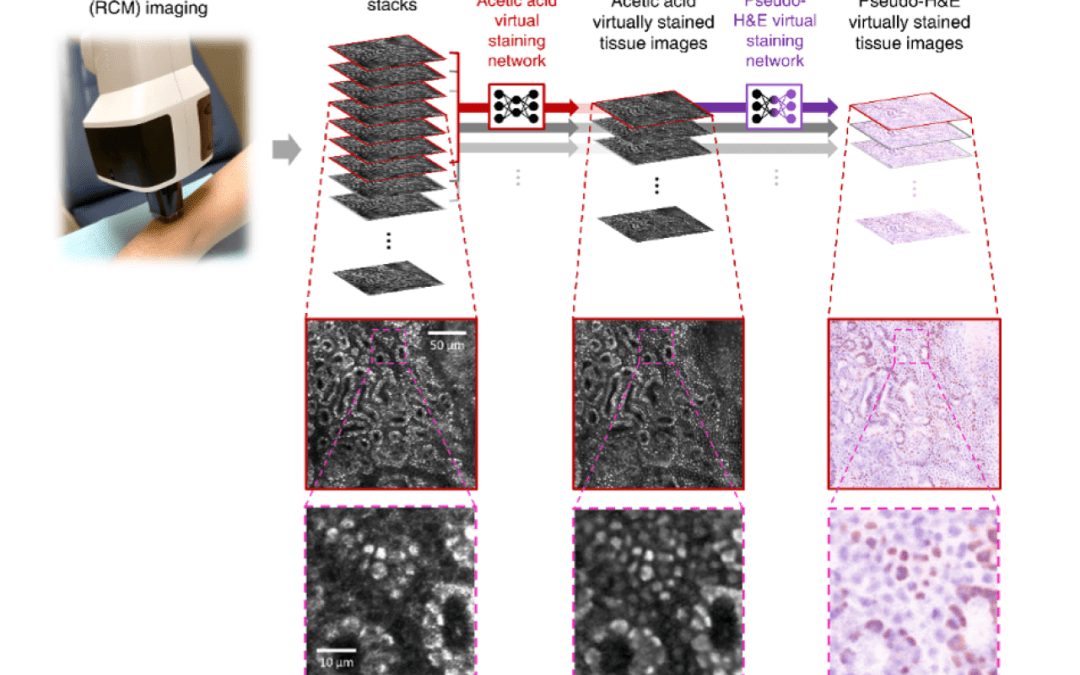
New imaging technology developed by UCLA research team may reduce need for skin biopsies
Instead of surgically removing a sample of skin, sending it to a lab and waiting several days for results, your dermatologist takes pictures of a suspicious-looking lesion and quickly produces a detailed, microscopic image of the skin.
This could become routine in clinics, the result of a new “virtual histology” technology being developed by researchers at the UCLA Samueli School of Engineering and the David Geffen School of Medicine at UCLA, according to today’s article in Light: Science & Applications, a journal of the Springer Nature Group. Histology is the study of the microscopic structure of tissues.
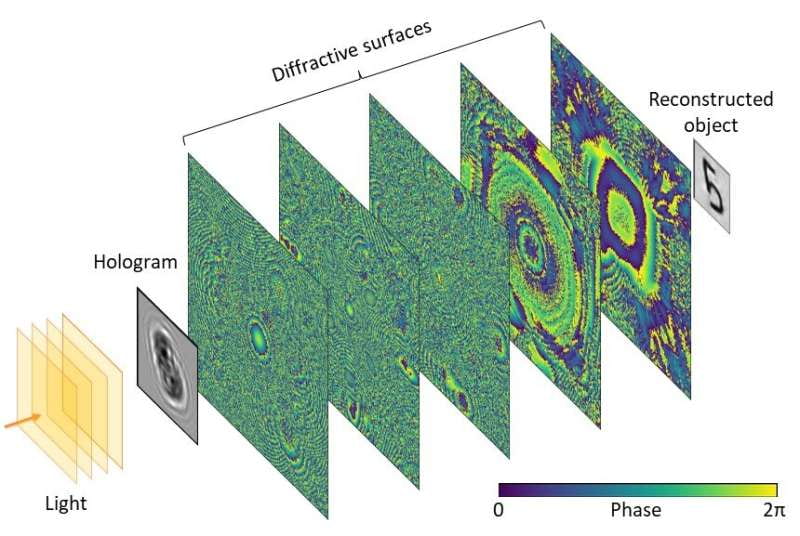
Diffractive optical networks reconstruct holograms instantaneously without a digital computer
In a recently published article in ACS Photonics, Professor Aydogan Ozcan and graduate student Sadman Sakib Rahman from the California NanoSystems Institute (CNSI) and the Electrical and Computer Engineering Department at the University of California, Los Angeles (UCLA) presented a novel approach for computer-free, all-optical reconstruction of holograms using diffractive networks.
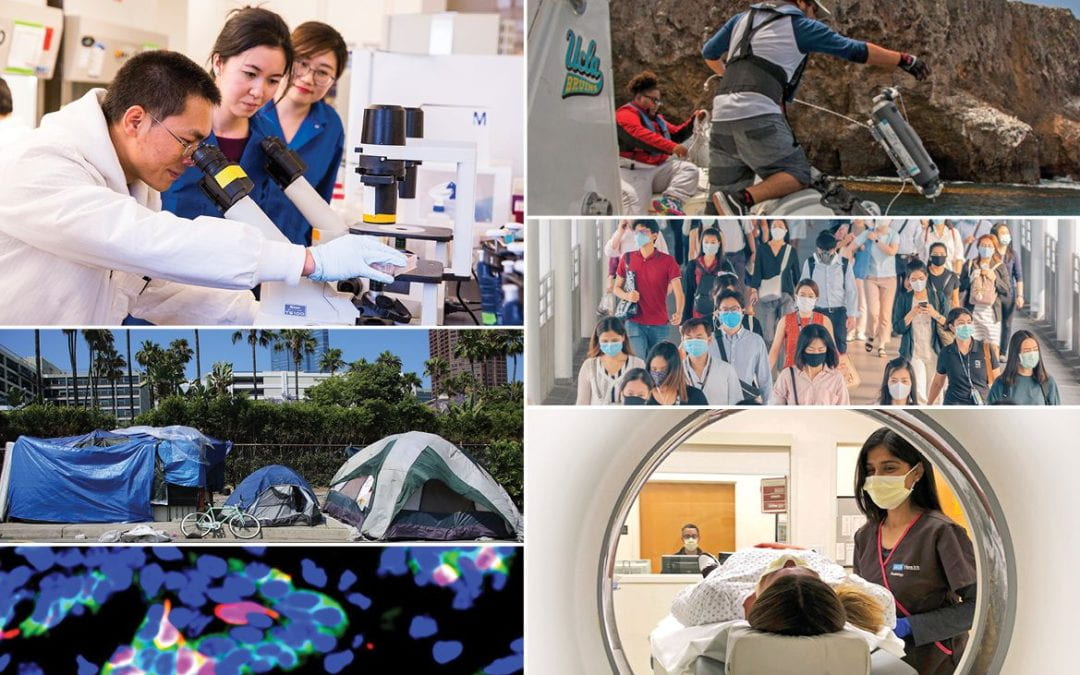
43 UCLA scholars among most highly cited researchers for 2021
In its latest annual list, Clarivate Analytics names the most highly cited researchers — the scholars whose work was most often referenced by other scientific research papers in 21 fields in the sciences and social sciences. The researchers rank in the top 1% in their fields, based on their widely cited studies. The 2021 list is produced using research citations from January 2010 to December 2020.
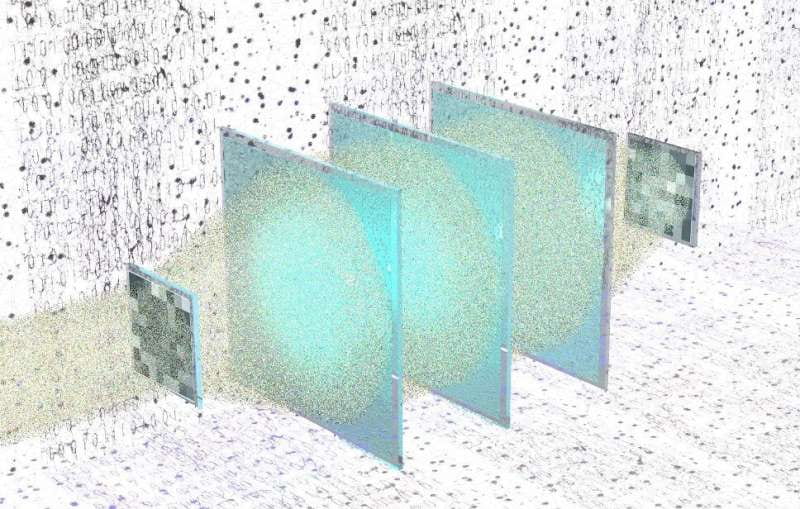
All-optical processors could compute any linear transformation, machine learning reveals
Researchers in the US have shown how all-optical processors could be used to carry out a range of linear mathematical transformations, including Fourier transforms. Using machine learning techniques, Onur Kulce, Aydogan Ozcan and colleagues at the University of California, Los Angeles, generated the blueprint for set of diffractive surfaces that can be used to produce specific optical outputs from any arbitrary input.
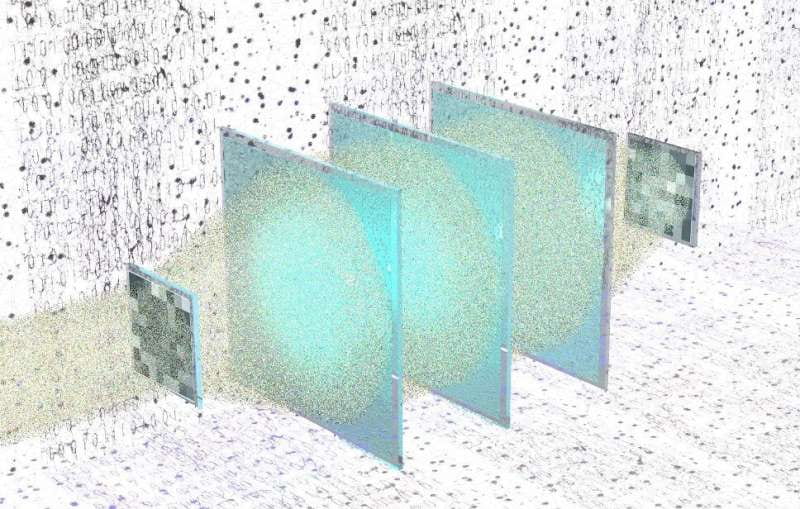
Light computes any desired linear transform without a digital processor
In a new paper published in Light: Science & Applications, a team of optical engineers, led by Professor Aydogan Ozcan from the Electrical and Computer Engineering Department at the University of California, Los Angeles (UCLA), U.S., and co-workers have developed a deep learning-based design method for all-optical computation of an arbitrary linear transform.
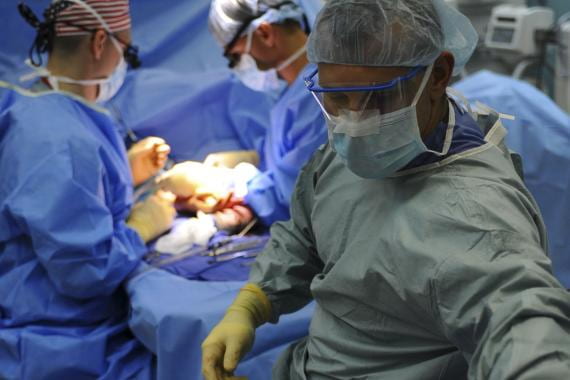
AI enables much faster pathology for life-or-death interventions
After training deep neural networks on around 4,000 slide images from around 40 biopsied kidney patients, UCLA engineers have virtually re-stained tissue images for speedier high-accuracy diagnostics than a human histotechnologist could support.
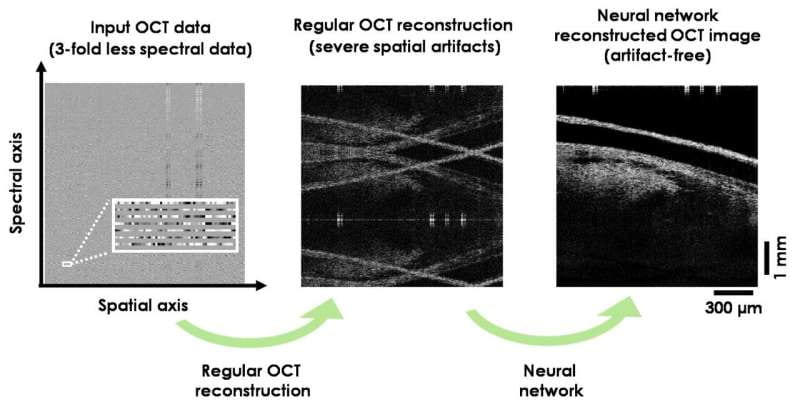
Deep Learning-Trained Neural Network Reconstructs OCT Images
A team of UCLA and University of Houston (UH) scientists, led by Aydogan Ozcan in collaboration with Kirill Larin, used deep learning to train a neural network to rapidly reconstruct OCT images using undersampled spectral data.
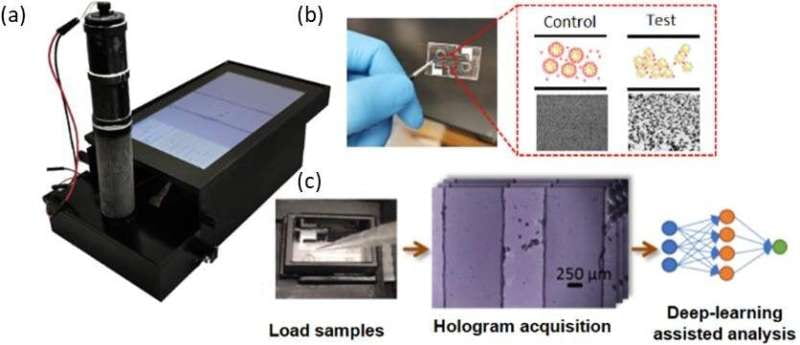
Deep learning and holography create a better point-of-care sensor
In a new paper published in Lab on a Chip, a team of scientists from the University of California, Los Angeles (UCLA) has developed a rapid and cost-effective particle agglutination based sensor that is powered by holographic imaging and deep learning.
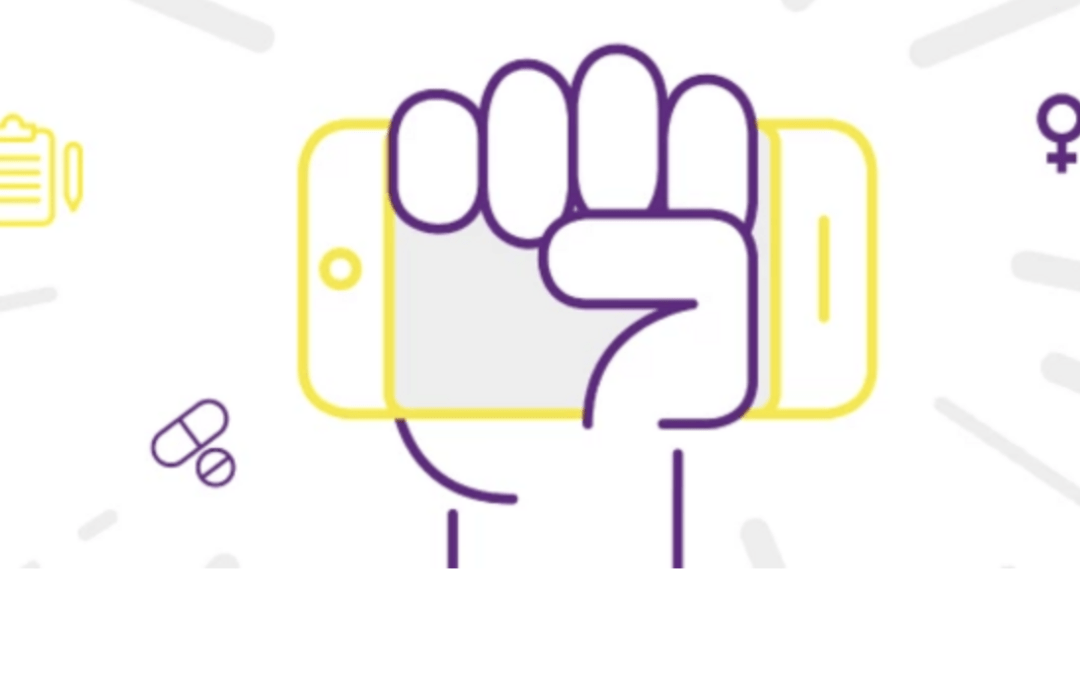
Empowering the Analytical Scientist in Everyone
Could the general public prove to be key in the next great phase of analytical chemistry? Say hello to citizen science!
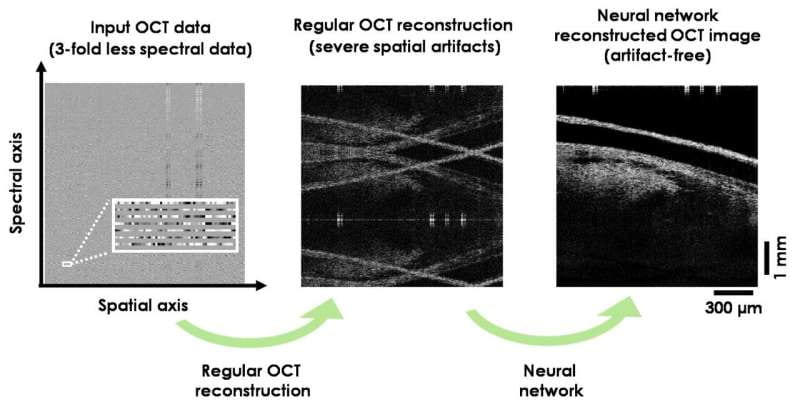
Deep learning improves image reconstruction in optical coherence tomography using less data
In a new paper published in Light: Science & Applications, a team of UCLA and University of Houston (UH) scientists have developed a deep learning-based OCT image reconstruction method that can successfully generate 3D images of tissue specimen using significantly less spectral data than normally required.

Artificial intelligence delivers faster holographic imaging
US-based researchers, led by Dr Aydogan Ozcan of the Bio- and Nano-Photonics Laboratory, University of California Los Angeles, have developed a holographic phase retrieval method to reconstruct microscopic images of samples some 50 times faster than existing methods.
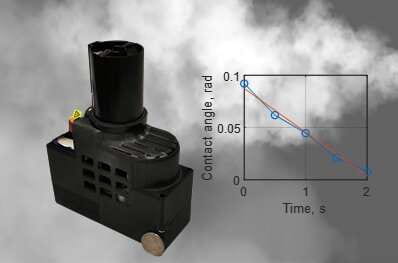
Rapid measurement of aerosol volatility using a deep learning-based portable microscope
https://phys.org/news/2021-06-rapid-aerosol-volatility-deep-learning-based.html
For older press releases, please visit this link